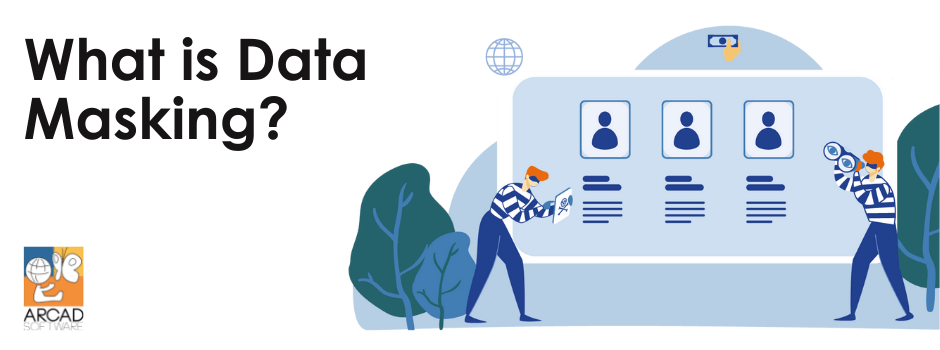
The amount of data produced around the world every day is growing continually. In 2010, the world produced about two zettabytes of data (one zettabyte is equivalent to one billion terabytes, or one thousand billion gigabytes). By 2020, this figure has increased almost 25-fold. And this exponential growth is expected to continue. By 2035, a study commissioned by Statista estimates that the world will have produced 2142 zettabytes of data. This increase is partly due to the arrival of the Internet of Things (IoT) and new technologies such as 5G, which open up new horizons for data. However, this massive influx of data leads to increased exposure of personal data and users' privacy can quickly be put at risk. To solve this problem, it is imperative to anonymize data and implement solutions such as data masking...
1. Data Masking: a key solution for all companies
By definition, Data Masking is a technology to “prevent the manipulation of personal or identifying data by giving users fictitious (but realistic) data instead of real data”. This technique guarantees the confidentiality, availability but also the integrity of the data for users and for companies. This method of data processing therefore allows organizations to keep datasets usable but no longer containing any “accurate” data, so as to protect the privacy of users.
2. “Data Substitution”, an example of a process to anonymize data
In simple terms, data masking allows us to retain realistic data while obfuscating any personal elements. For example, in a survey to find out the preferred color of a group of people based on their age, we would have the following information: first name, age and preferred color. For this example, data masking on this dataset would allow the first name to be changed to a fictitious name. This process, known as “Data Substitution”, makes it possible to keep the preferred color according to the age of the person while eliminating any possibility of identification. Although the first name is no longer accurate, this change allows the study to be completely anonymous and to meet the criteria for the protection of personal data. As explained in the definition of Data Masking, this technique creates “fictitious (but realistic) data instead of real data”.
In short, on the scale of a dataset containing several hundred of entries, the fictitious data created by this Data Masking method would give a representative result of the initial dataset, while making the identification of an individual within the group studied completely impossible. A simple, yet functional process, allowing to meet the GDPR standards while keeping quality data...